Spotlight on Technology: Adoption of Artificial Intelligence in Medical Devices
Navigating Medical Device Industry Trends by Adopting the Digital Thread
Welcome back to our blog series where we highlight the most impactful trends in the medical device industry across four dimensions: technology, business, patient care and compliance. Each post will address how the digital thread can help companies embrace these trends to improve business outcomes.
Blog 1: Navigating Medical Device Industry Trends by Adopting the Digital Thread
Blog 2: Spotlight on Technology: Adoption of Artificial Intelligence in Medical Devices
Blog 3: Technology Trends Continued: The Growth of Connected Devices and the Internet of Medical Things
Blog 4: Medical Robotics: The Future is Now
Blog 5: AI's Growing Influence on the Medical Device Company’s Value Chain
In our previous blog on trends in MedTech, we touched on how adoption of the digital thread is the next frontier for advancing the medical device industry. We are already witnessing breakthroughs in many uses of artificial intelligence (AI) and Machine Learning (ML) in devices, including medical robots, patient apps, diagnostics and smart connected devices, also called Internet of Medical Things (IoMT). AI is perhaps the most profound mega-trend that will influence the industry.
Broadly, AI refers to the use of artificial intelligence. ML is the training of models (think of these like artificial neural networks or brains) and can be considered a subset of AI. In this blog, we will refer to both with the combined term, AI/ML.
The advancement of AI/ML in everyday life is impacting us exponentially with smart homes, self-driving cars and large language models like OpenAI GPT-x and Google’s Gemini. It is suddenly around us in subtle and not-so-subtle ways.
There are many ways AI/ML is affecting the MedTech industry. This includes:
- Integration of AI/ML within the medical device itself
- Utilization of AI/ML for the development and design of new or enhanced iterations of medical device products
- Application of AI/ML in the processes of manufacturing or servicing the device
In this entry, we explore the first of these three. Later in the series, we come back to the second and third concepts on this list.
The Explosion of Devices with AI/ML
One of the initial uses of artificial intelligence in clinical settings was for early detection of atrial fibrillation. In 2014, the Food and Drug Administration (FDA) approved AliveCor's mobile application, Kardia, for this purpose.
Since then, the FDA has seen an increasing number of marketing submissions and pre-submissions for devices utilizing AI/ML and expects this trend to continue. To help navigate the complex landscape of digital health, the FDA has established a Digital Health Center of Excellence. This move shows the FDA’s recognition of the immense potential of AI as well as their support of it in the industry.
It is also worth noting that the FDA has published 10 Good Machine Learning Practices for medical devices with embedded AI/ML.
Here are a few examples of AI/ML embedded in devices:
- Image analysis: Medical imaging devices generate large amounts of data that can be hard for a human to interpret. Newer AI/ML models are getting even better at identifying patterns that may indicate disease, such as detection of tumors.
- Monitoring and diagnosis from IoMT Products: AI/ML is now being used to analyze data from wearables, such as heart monitors, sleep apnea or smart orthopedic implants to identify patterns that may indicate the presence of disease. This is useful in the detection and diagnosis of diseases and conditions, and the understanding of the efficacy of a surgery (as in the case of an orthopedic implant).
- Personalized medicine: AI/ML is being used to analyze data from medical devices to create personalized treatment plans for individual patients. This can help to ensure that patients receive the most appropriate treatment for their condition.
- Natural Language Processing (NLP): Medical devices can have significant potential to leverage NLP to understand natural language inputs and provide relevant outputs. This can facilitate communication between patients and medical devices, making the interaction more intuitive and user friendly. Undoubtedly large language models will soon be integrated into medical assistant devices. It’s not hard to imagine examples where NLP medical data (scrubbed for privacy) will be incorporated along with data from medical devices to enhance Real-World-Data capture.
- Research Assistance: Large language models are already being used in clinical research, for example to better match patients to clinical trials.
The FDA publishes a list of medical devices with AI/ML embedded. It’s currently dominated by diagnostics, but the range of applications is growing.
As of 2023 this number is clearly rising rapidly per Figure 1 while Figure 2 illustrates categories of devices where Machine Learning and AI/ML is utilized:
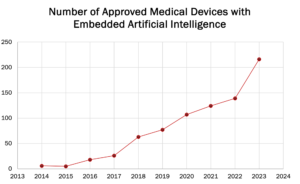
Figure 1: The rapid rise of AI/ML within medical devices
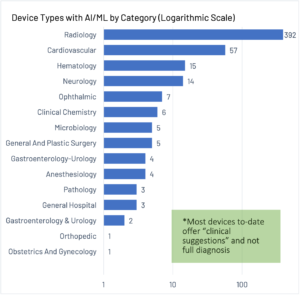
Figure 2: The categories of devices with AI within medical devices
Regulatory Framework for Machine Learning in Medical Devices
FDA Risk Based Framework
The FDA has established a risk-based framework outlined in both pre-market and post-market guidance documents regarding the use of ML in medical products. This framework is available publicly and lists all medical devices that incorporate some form of ML.
In 2023, the FDA introduced specific guidance on integrating a Predetermined Change Control Plan (PCCP) for ML within medical devices. This guidance addresses both automatically and manually implemented changes to models, facilitating a process where modifications can be made without additional approval. The PCCP is structured into three key components:
- Description of Modifications
- Modification Protocol
- Impact Assessment
These elements are designed to streamline the management of ML modifications, ensuring safety and compliance while enabling innovation.
FDA Progressive Commitment
The FDA is clearly committed to enabling the use of AI/ML in medical products (including those that continuously learn within a safe set of parameters) and bringing more structured regulation.
Relevance to Digital Thread
Organizations can use AI/ML to optimize the performance and capability, safety and efficacy of medical devices. The FDA has left room to allow AI to learn and adapt within a safe set of parameters as devices are used without resubmitting through a regulatory process with PCCP.
Businesses will need to advance their digital thread backbone to incorporate AI/ML in the way the FDA envisions which is a Total Product Lifecycle approach (TPLC) already discussed in the first post in this series.
The following will need to be well-controlled and digitized:
- Management of the product (including software and model) design and development
- The PCCP
- Manufacturing, setup, monitoring of data
- Service of the Device
It is simply too complex to handle these kinds of requirements without a well-controlled set of interconnected capabilities supported by modern platforms which ideally would include the following minimum list:
- ML-Ops: manages the development, compression and maintenance of the AI/ML models
- Application Lifecycle Management (ALM): manages requirements, risk, test and software through its lifecycle
- Product Lifecycle Management (PLM): controls as many as 60 aspects of the digital thread, but most notably the Design History File, Device Master Record and Change Control Process
- Regulatory Information Management (RIMs): controls submissions
- Quality Management System (QMS): controls the management of quality incidents
- Manufacturing Execution Systems (MES): controls the production of devices and the Device History Record
- Smart Connected Product (SCP)/Internet of Medical Things (IoMT): latform which connects the device and gathers Real World Data
- Data Lake: allows for robust Data Analytics of data generated by these systems including descriptive, diagnostic, predictive and prescriptive modes.
The interconnectivity of processes and data are significant. Changing one aspect of the design will pull on many other elements even more so than ever before. Capabilities within a mature digital thread will allow companies to manage this information in a way that is efficient and compliant.
Further, the capture of Real-World Evidence (RWE), which will require companies to gather data from devices in secure and robust methods, adds to the increased importance of getting the model well managed and under control.
Figure 4 is a summarized depiction of the digital thread across the total product lifecycle and the broader value chain and illustrates just some of the key aspects of the AI Model and it’s PCCP and collection of RWE that can be automated and managed across each part of the digital thread:
Figure 4
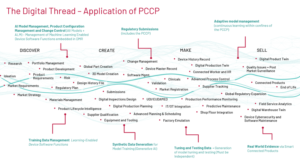
Emerging Frontiers
Model Compression and Edge Computing Opens New Frontiers
Breakthroughs in model compression make for easier portability and consumption at the edge. This is especially important because not all hospitals and clinics are willing or able to permit the IoMT or “connected” concept and there will be many instances where an internet connection is either not fast enough (too much latency) or just unavailable. It is worth noting that a mature ML-Ops facilitates the automation of various stages in the machine learning pipeline, including the integration of model compression techniques. This automation can include the optimization of models for deployment, where model compression plays a crucial role.
Multi-Model Use Cases
Multiple AI models are being combined to further drive patient outcomes, and many small advancements in these large AI models are being developed at a breakneck pace but aggregate into major advancements in the field. AI is further being incorporated into the broader clinical workflow and there are many variants.