Extend equipment health, prevent failures and improve reliability with IoT connectivity and AI.
Companies in the industrial sector face continuous pressures to boost operational efficiency, reduce downtime and extend equipment life. Traditional maintenance methods often result in costly unplanned failures or excessive upkeep. Aging infrastructure, increasingly complex machinery and harsh operating environments exacerbate the challenges to maintaining reliability.
Predictive maintenance offers a solution, and is a proven driver of measurable business value. By leveraging machine data to predict maintenance needs and prevent equipment failures, this proactive approach reduces total cost of ownership, boosts asset availability and enhances worker productivity and safety. Predictive maintenance enhances operational efficiency, reduces costs and minimizes risk.
We partner with industrial operations to deploy predictive maintenance capabilities, using machine data and established data science principles to deliver results as soon as 8-12 weeks.
Real Results
- Enhance asset availability by 10-30%
- Improve worker productivity by 10-15%
- Lower total cost of ownership by 8-40%
- Prevent catastrophic failures and improve worker safety
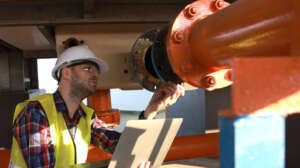
How We Help Companies Enable Predictive Maintenance
Condition Monitoring
Schedule maintenance, visualize results and take timely action to prevent failures:
- Advanced Sensing
- Edge Computing
- Machine Learning
Operations Management
Monitor asset performance, plan maintenance tasks and visualize results, enabling timely action to preempt failures with:
- APM
- CMMS
- IIoT
Reliability Centered Maintenance (RCM)
Ensure investments in predictive maintenance focus on the right assets, yielding tangible value with:
- Reliability Growth Modeling
- Opportunity Prioritization
- Technology Analysis & Design
- Change Adoption
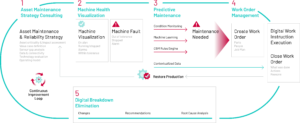
High-Value Predictive Maintenance Use Cases
Predictive maintenance is a key priority for global manufacturers, our solutions address critical challenges:
Process Bottlenecks
In discrete and hybrid production sectors like tires, semiconductors and automotive, anomaly detection prevents downtime in critical processes, increasing throughput
Remote Operations
In process industries and OEM sectors where breakdowns can trigger costly delays, predictive models anticipate failures and optimize field service routes, ensuring critical assets are prioritized and reducing the likelihood of downtime
Complex Manufacturing Processes
In industries where standard condition monitoring methods like vibration analysis are insufficient, deploying complementary systems such as machine vision and robotic inspection detect deviations, minimizing downtime and ensuring product quality
Safety-Critical Components
In safety-focused sectors like mining, predictive maintenance ensures the reliability of crucial assets like ventilation systems to protect workers
Our Approach
Our projects typically begin in one of two ways: with a clearly defined opportunity and value case, or with a program charter seeking value. Overall program timeline varies based on the starting point and end target of the client, but we always seek to bring solutions live in production and create value within eight to twelve weeks of any project.
The Kalypso and Rockwell Automation Difference for Predictive Maintenance
Comprehensive solutions integrating hardware, software and services
Extensive portfolio of platform-based tools
Our solutions offer seamless edge-to-cloud connectivity for condition monitoring and operations management, with the flexibility to add capabilities as needed.
- Innovative Signal-Based Monitoring: Our FactoryTalk Analytics GuardianAI analyzes Variable Frequency Drive (VFDs) electrical signals for comprehensive monitoring or the entire production process without needing additional sensors.
- Edge Computing and Machine Learning (ML): Machine learning models deployed at the data source provide real-time insights while minimizing network and storage demands, and pre-built ML models enable rapid solution implementation without extensive data collection.
- Comprehensive Asset Monitoring: Beyond traditional methods like vibration analysis, we employ cutting-edge technologies like machine vision and robotics, integrating seamlessly with various management platforms like Fiix, FactoryTalk DataMosaix and Plex Asset Performance Management, for a complete view of asset performance.
Strategic partnerships
We collaborate with leading Industrial DataOps, IoT, AI, and Cloud providers, seamlessly integrating their technologies with Rockwell’s sensing, controls and analytics solutions. This allows us to deliver best-in-class, integrated architecture solutions that combine shop floor practicality with enterprise-scale performance.
Rapid deployment and long-term success
Our team combines industry, data science and consulting expertise to quickly identify high-impact areas, deliver measurable value in weeks and scale results through a programmatic approach.