The Autonomous Enterprise: Principles of Autonomous Systems
Let’s start with the word “autonomous”, which means “self-governing”. Autonomous manufacturing is the manifestation of “self-governing” systems at every step of the manufacturing process. These systems derive their autonomy from data-led decision-making models that enable them to reliably adapt their behavior in response to dynamic environments during operation without any manual intervention.
While the control algorithms and feedback loops are an integral part of an autonomous system, it is important to understand the distinction between automation and autonomy. The fundamental difference is that an automation system is explicitly programmed to perform tasks in the absence of human intervention, while an autonomous system can learn how to perform tasks on its own and even adapt to changes in a process or environment.
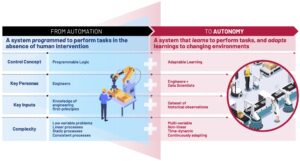
Put simply, the autonomous system replicates the cognitive ability of humans to learn, make decisions, take action against those decisions, and continuously improve. You are likely familiar with autonomous systems such as self-driving cars, autopilot on aircraft, and other areas where a complex system is making decisions and actuating those decisions mostly independent of human operations. These concepts are analogous in the manufacturing industry.
Principles of Autonomous Systems
Autonomous systems—from household thermostats to autonomous vehicles — share four common principles:

Autonomous Manufacturing Maturity Spectrum
Autonomous manufacturing capabilities are a game-changer for industrial clients and have the potential to unlock significant business value. They eliminate or repurpose the need for human decision-making, especially when the volume, variety and velocity of those decisions are yet times beyond human capabilities.
Achieving autonomy requires establishing capabilities, all the way, from observation, explanation, and inference to decision making and action. However, a majority of the players in the Industrial AI domain today are focused on just making inferences and predicting potential outcomes. Achieving autonomy and truly realizing significant business value, requires maturing the capabilities all the way to the prescriptive (decision) and autonomous (action) stages of the maturity spectrum. We strongly believe our experience with this is what differentiates Kalypso from where the market is today.
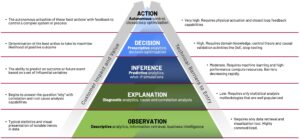
Also, this journey is not without its own set of unique technical barriers to entry. Establishing these capabilities requires strong, multi-disciplinary skillsets with industry-specific domain knowledge, data science, advanced control theory, IT-OT architectures, and process subject matter expertise to derive causally actionable data and models. Most importantly, this requires expertise and focus to achieve autonomous capabilities with utmost and keen attention to the safety, security and stability aspects of the process.
With the right skillsets in place, autonomous system capabilities can be manifested utilizing a methodology called “Closed-Loop Optimization”, which incorporates elements of supervised learning and reinforcement learning. A continuous reinforcement loop of control adjustments aimed to reduce variability and optimize target process variables enabled by a robust digital twin of a physical system composed of engineering first principles and data-derived machine learning models.
General Architecture for Closed-Loop Optimization
The diagram below represents the general architecture for autonomous manufacturing. It starts with the development of a digital twin of a physical system. A digital twin is a digital representation of a physical process or machine that replicates its behavior as it undergoes changes in its environment or instructions. Oftentimes most of the system’s behavior can be codified into logic based on the engineer’s experience. However, there will likely be a gap between what can be derived out of first principles and how the system performs. That gap is filled by data scientists who derive analytical models based on data that describes the historical behavior of a system.
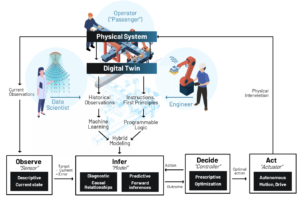
A critical element of the system is observations that are obtained from sensors in the line. Sensors collect raw data, which is transformed and compared to better understand how specific variables within the system interact. These relationships are then codified into predictive models that can be used to evaluate different scenarios. In the prescriptive optimization phase, the model determines which adjustments or manipulations to the physical system are predicted to yield the most desirable result. Once the model is running in a live environment, it can provide predictions and recommendations.
For example, the model may sense that a critical process parameter is drifting above or below an ideal set point. Based on learnings from what causes this variation and what actions can solve it, the model can evaluate a very large array of possible actions to take and recommend the action that will drive the best outcome.
These actions are applied by a control system, a drive, or something that physically intervenes according to control system instructions. This continuous process consistently strives to improve the outcome of the system or bring it to a desirable state.
To understand these basic principles of autonomous systems, consider this scenario in which a human driver approaches a stop sign. From observation to inference, decision, and action, the driver’s response to the stop sign perfectly illustrates the way a system of closed-loop optimization would perform.
What is the value?
- Better decisions that lead to more desirable outcomes and eliminate undesirable outcomes.
- Faster and more numerous decisions that enable more fluid control over a complex process or system.
- Autonomous decision making that eliminates or repurposes the need for manual effort.
In a business context, this leads to better planning, scheduling and resource allocation decisions, reductions in resource and raw material inputs, faster rates of production, higher levels of quality and yield and greater capital asset efficiency.