How to Use AI to Augment Field Service Operations in Life Sciences
Even before the current crisis, life sciences companies were adopting artificial intelligence (AI) into daily service activities. As companies adjust to the reality of increased remote engagements, they can accelerate the trend towards connected field operations.
AI augments the service engineer, allowing him or her to make more informed decisions, better predict outcomes such as personnel scheduling and parts shortages, and identify and respond to product quality issues before they become critical for end users.
In a survey conducted in conjunction with Astea, Field Service Medical (FSM), and WBR Insights1, the Internet of Things (IoT), AI, and preventative maintenance accounted for 63% of the top three items executives consider when purchasing a Field Service Management platform.
Here is how we see AI impacting day-to-day operations, both through and after the recovery.
Drive Out Complexity
It has been well documented that companies are losing skilled service personnel at a faster rate than they are backfilled, largely because baby boomers and Gen Xers are retiring, and millennials are not interested in field service work.
In a report by Manpower Group, 70% of the companies interviewed said they expected a skills shortage over the next 10 years.2 In addition to the loss of talent and knowledge, the sheer complexity of modern machinery makes it difficult for subject matter experts to keep abreast of best practices. Tools based on AI must be used to augment the SMEs’ capabilities and bridge the skills gap.
The growing complexity of modern systems increases multi-mode failures which describe how many causes of failure act together to affect the performance of a system. With such complexity, it is almost impossible for one person to fully understand the intricacies of equipment operation, and historical service practices that used procedural lists and rules of thumb are ineffective in diagnosing root cause analysis.
I recently witnessed a hidden problem while helping diagnose a highly complex piece of equipment using AI. After the issue was resolved, root cause analysis identified two items:
- The problem was severe enough that the organization wanted to ensure new models used in the field were fixed too. But as service engineers were deployed to fix the issue, they discovered the manufacturer of the equipment had already addressed the situation without updating them. Therefore, any rules which might have been developed to address this specific failure would already be obsolete on newer models.
- Risk analysis indicated the severity was high, the mechanism for detection difficult, and the likelihood low (which is becoming the norm as machines become more complex). The sheer number of rules needed to catch these potential failures would require months of effort and become outdated with any new software and/or hardware revisions.
With AI, companies can quickly evaluate and visualize complex data sets to help uncover complex failure patterns never observed before. Artificial Intelligence augments the knowledge of highly skilled SMEs and guides them towards identification of multi-mode failures.
Significantly Improve Customer Satisfaction
For many companies, it used to take several hours to review service calls, support tickets and voice mails from angry customers to understand where the hottest fire was.
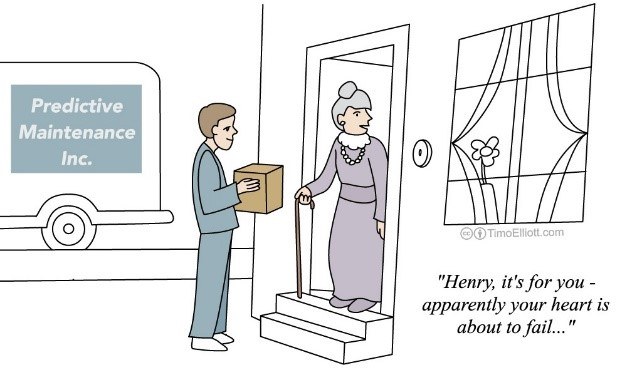
Today, connected devices provide enriched sensory information like operating hours, internal operating temperatures, fluids dispensed and diagnostic alarms. This means service organizations can apply AI algorithms to predict when a failure will occur.
Customer satisfaction ratings dramatically increase because technicians can provide guidance on impending breakdowns and organize maintenance around the customer’s schedule.
In one example, a predictive maintenance package alerted the manufacturer to a tube failure of a CAT scanner seven days before it occurred, saving hospitals $100k/catch in replacement costs (not including labor and downtime costs).3 Moving from reactive to predictive maintenance can lead to 20-40% reduction in downtime and 15-30% reduction in maintenance costs.4 These AI-driven preventive maintenance alerts have a significant impact on reducing the cost of poor quality.
Optimize Spare Part Inventory (with a Flock of Seagulls?)
Spare parts management and optimization is important because it creates a more efficient process of identifying both availability and location of spare parts. Predictive maintenance algorithms identify when something is going to happen (to a specific model, in a specific region, with a specific failure mode), but before the technician gets in the truck, she is going to need to procure a specific part to fix the client’s device.
The question becomes: Is that part on-hand?
Although it might appear less expensive to simply buy and keep parts in stock, imagine the costs if the enterprise is running global operations. The costs to store parts in multiple field locations becomes expensive and the strategy quickly becomes impractical.
For the answer we can turn to nature and the majestic seagull. With advanced genetic algorithms and particle swarm optimization (PSO) techniques that mimic the behavior of flocks of birds, we can optimize the availability of stocked parts.
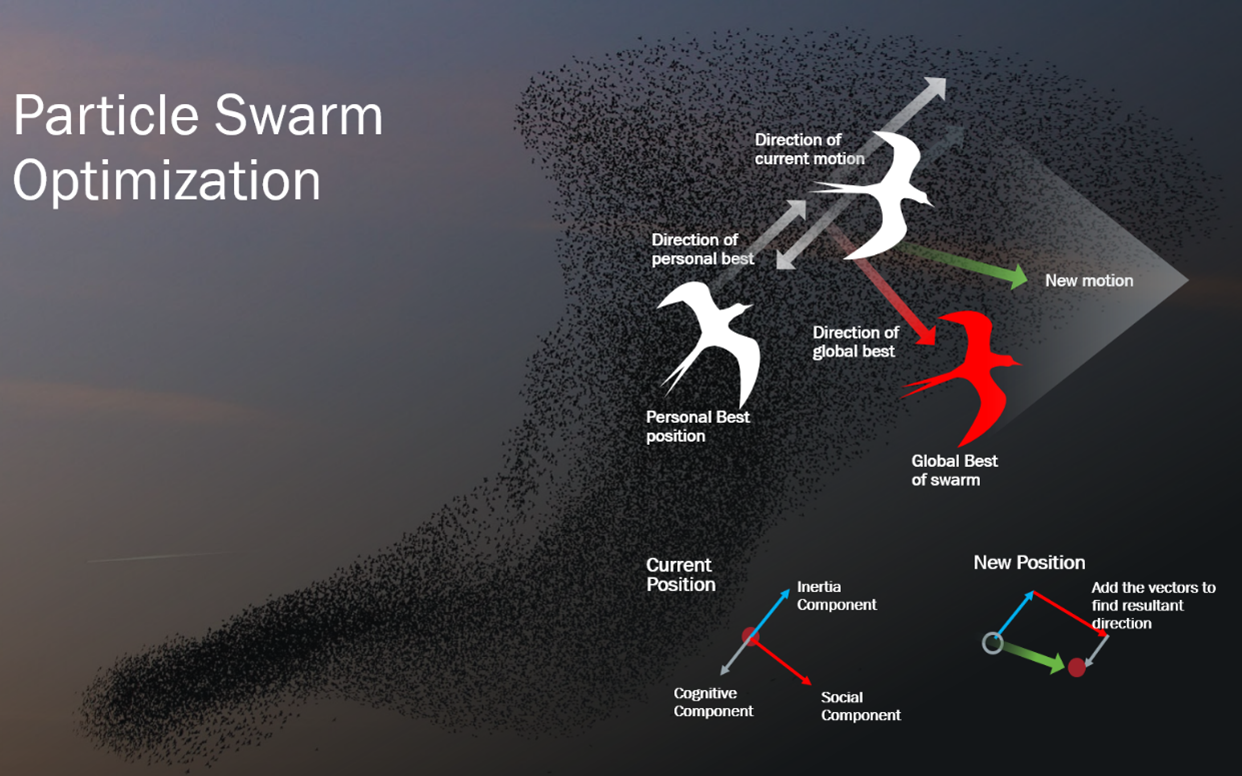
Imagine a swarm of birds, such as a flock of seagulls, seeking out their next meal. Many behavioral, social, and historical inputs exist which lead the group to finding the optimal solution given their current state.
If you consider a single seagull, it will try to seek food in one direction based on historical learnings and memory. But when you have the social component of the group, you capture the combined history of the flock, leading the swarm in the optimal direction. The flock gets to their dinner quicker than if all that existed was the information from a single individual.
These high-end optimization techniques can be applied to everyday service situations as well, seeking input from sources of field asset health data, cost data, service engineer availability, etc. By automatically executing millions of combinations and constraints, algorithms can generate optimal maintenance schedules along with part quantities that will minimize inventory carrying costs or enterprise impact costs. These AI-based optimizations ultimately allow companies to operate optimal predictive maintenance schedules with spare parts inventory, where the right parts are available in the correct quantities at the required locations.5-8
Achieve Efficiency via Intelligent Scheduling
Skilled personnel are retiring at a rate faster than positions can be filled.9 This forces organizations to maximize all available resources and overbook appointments to reduce overtime and unnecessary costs. With AI, organizations can put their most skilled people in the right locations at the right time.
This helps companies:
- Reduce travel times, fuel costs, and vehicle maintenance
- Ensure work orders and technician skillsets match, thus reducing the cost of lost appointments
- Avoid costly Service Level Agreement (SLA) penalties
Personnel scheduling has historically been a very manual, ad-hoc process that requires evaluating incoming jobs, allocating appropriate resources, and performing rudimentary sequencing and scheduling. There are several inputs in this equation including technician availability, the type of service required, device-specific training, and the expected service time per issue, which make scheduling a highly complex optimization problem for anyone.
And even once a schedule is defined, employees who are unwell, stuck in traffic, or delayed at other sites can cause them to start the process over again. There are too many variables for any single person to adjust all the levers, so an advanced analytics approach can help efficiently optimize the scope.
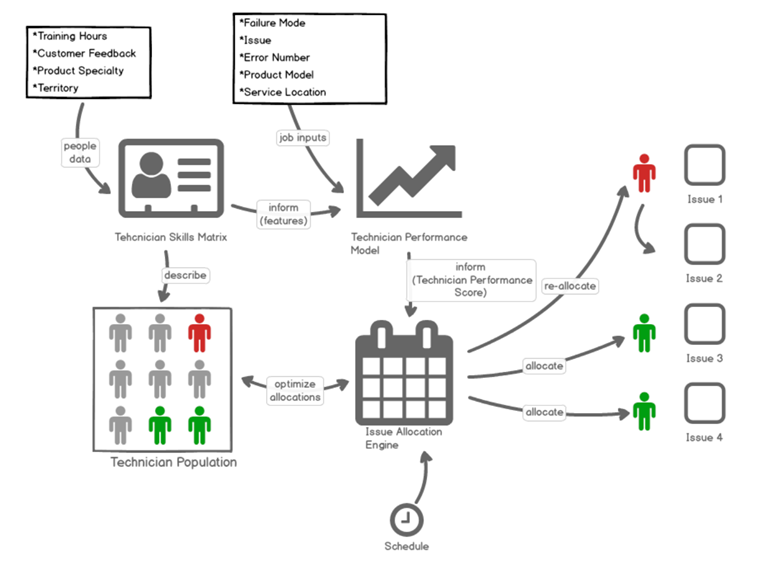
This image proposes a method where inputs from a digital skills matrix describe each technician in a workforce population. Skills including training hours performed, customer satisfaction metrics, product specialties, territory, etc., are used to build a technician performance model.
The model provides input to the Issue Allocation Engine which examines the entire population of technicians and work orders (using the Hungarian Algorithm for example) and optimizes the right set of resources based on the job inputs (failure mode of equipment, model type, service location, job duration).
With this type of optimization algorithm, we’ve seen our clients service organizations improve productivity from 10-20%.
Getting Started with an MVP Approach
There are clear benefits for companies that connect devices using AI and machine learning. But when it comes to getting started, there are a few key things to keep in mind.
The most important thing companies can do is consider current business challenges and apply an AI lens. When AI is used to help solve real, compelling business challenges, companies can realize real value quickly with a minimim viable product (MVP) approach.
The MVP approach is based on the idea that depth of value beats breadth of application. Instead of focusing on building a broad base of foundational AI capabilities generalized across multiple use cases, the MVP approach builds a full stack of capabilities and value focused on a narrow, high-priority use case.
This full stack starts with foundational connectivity and data models, and also includes descriptive analytics, root cause analysis, predictive modeling, and all the way up to prescriptive modeling and optimization.
When value is realized for the high-priority use case (ideally linked to a significant business challenge), companies can then scale for additional use cases.
Successful service companies are thinking about AI now. Part of adapting to the new normal will be adapting strategies to put remote work first. This might come from an MVP around predictive maintenance of failures, which would allow technicians to be onsite for only the most critical of issues. It may come from improved technicain scheduling, minimizing windshield time while maintaining or exceeding customer satisfaction metrics during this challenging time.
AI doesn’t have to be a mystery. Avoid black box approaches based on proprietary, ‘behind the scenes’ data sets and analysis. Instead, provide business context and a strong value case, and apply an agile MVP approach. You’ll enable platforms and methodologies that will sustain ROI for years to come.