Industry 4.0 and the Role of Industrial Data Management
Unlock the Full Potential of Your Data
The term Industry 4.0 has been around for over a decade and originally focused on the integration of digital and manufacturing technologies. The scope of the term has since evolved to include the manufacturing application of technologies such as the Internet of Things (IoT), AI and advanced analytics, robotics and augmented reality.
The rise of Industry 4.0 and Internet of Things (IoT) has also driven the increased need for, and emphasis on, the management of data within manufacturing, also known as operational technology (OT), and its integration with corporate data or information technology (IT), collectively referred to as IT/OT convergence.
This convergence, however, may initially present itself as a divide to many companies due to the different perspectives, priorities and skills between IT and OT, as shown below.
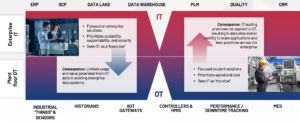
Without paying attention to this divide, companies trying to deploy Industry 4.0 initiatives will continue to suffer from siloed data that lacks the necessary, but unassigned, context to yield full value.
Data Management 1.0
At roughly the same time companies started focusing on Industry 4.0 solutions, corporate IT saw a significant rise in data governance and strategy efforts to make IT data more meaningful, useful and ultimately valuable. With stronger adherence to architectural frameworks, deployment of cross-functional data governance bodies and processes, and implementation of management tools such as data catalogs, IT has been able to provide more robust and trusted enterprise level data.
Improvements in this area supported the rising demand for self-service reporting and analytics, reducing the load placed on IT to be a report generation function. Through the use of managed and trusted (and even certified) data sets, companies realized the benefits of putting more analytical capabilities directly in the hands of business users, spawning a new user category of citizen data scientists.
Next Up: Industrial Data Management
Now is the time to apply similar efforts to OT data, using Industrial Data Management (IDM) to break the IT/OT divide to maximize production efficiency through greater IT/OT connectivity, integration and use of managed and trusted data for real-time decision making.
Let’s first acknowledge the challenges and complexities of Industrial Data Management.
- Data is often housed in silos due to the prevalence of point solutions, with little to no support provided to improve data quality.
- Different data types and structures exist across a variety of point solutions that make interoperability difficult (e.g., time series, relational, unstructured and data referenced in engineering drawings).
The following diagram conveys a representative, functional IT-OT architecture in a manufacturing environment.
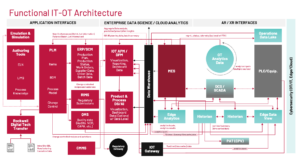
With IT and enterprise systems on the left and OT systems and the PLC automation layer on the right, high-level data flows characterize the integration that has evolved over the years. The boxes at the top for interfaces and analytics, along with the data warehouse in the middle, represent key functions in the architecture that get at part of the data management challenge. While the diagram highlights some functional applications in the IT space (e.g., PLM and ERP), the OT side emphasizes more of the data processing “engines”, such as PLCs, SCADA, historian and edge components.
While there are some common applications that use these engines, this is where you’ll see a very diverse landscape of solutions that manufacturers rely on, but make data management more challenging.
IDM is not just about implementing new software in the OT space; it requires a holistic approach that encompasses people, processes and technology for the various systems shown in the OT architecture, especially where data is aggregated in platforms such as an operational data lake.
Our field experience has produced three key recommendations. For success in IDM, manufacturers should:
- Develop a clear data strategy: Identify data to be collected, how it will be used, and who will be responsible for managing it. Align intended OT data processes with corporate IT capabilities where possible to streamline management efforts.
- Build a data-driven culture: Train employees to understand the value of data and empower them to use it to make better decisions. Treat data as another corporate asset, requiring maintenance and protection. Investments in data management will pay off if users understand the benefits of integrated and contextualized data.
- Invest in the right technology: Target IDM solutions that are scalable, secure, and interoperable with existing OT and IT systems. Understand how to deploy OT systems that not only improve production operations and real-time monitoring but also contribute to improved data management.
Real-World Examples of Industrial Data Management in Action
Industrial Data Management (IDM) extends beyond data collection and management. Its primary objective is to ensure data is accessible, meaningful, actionable and valuable. Let’s dive into manufacturing examples that demonstrate how IDM enhances both production and operations.
Quality Control
Make data more meaningful through integration with engineering documentation
In fabrication, laser scanners can capture precise measurements of finished parts. With IDM, the production system can compare these measurements against design specifications to identify deviations. This real-time quality control allows for immediate adjustments to the production process, reducing scrap rates and ensuring consistent product quality.
Real-time Production Optimization
Make data more useful through integration into closed-loop processing
Consider a filling line with sensors to monitor fill levels, line speed, and equipment performance. Going beyond traditional production monitoring and reporting, IDM aggregates this data, providing real-time visibility into the entire process. If a machine starts to slow down, a closed-loop system can automatically adjust the line speed to prevent bottlenecks, minimizing downtime and maximizing throughput.
Predictive Maintenance
Make data more valuable though predicting potential failures
Instead of reactive repairs, IDM enables predictive maintenance. By analyzing vibration, temperature, and pressure data from pumps and motors, Predictive Maintenance systems can predict potential failures before they occur. This allows maintenance to be scheduled proactively, preventing costly breakdowns and spills and extending equipment lifespan.
Many times, use cases like those above start at a simple level, collecting just the raw data necessary to support the target processes. However, low quality data usually yields low value results, barely offering visibility beyond highlighting that there may be an issue. With broader data sets, augmented through contextualization to increase the richness of the data, you can move beyond visibility to insights and action. Each use case highlights the ability to not only take action, but have that action triggered through use of the contextualized data.
The Benefits of Industrial Data Management
Ultimately, IDM uses people, process and technology to break down the IT/OT divide and provides rich and contextualized data that enables:
- Increased Efficiency: Streamlined operations, reduced downtime, and optimized resource allocation lead to significant efficiency gains. See other Kalypso Viewpoints related to Production Performance Improvement for more insights.
- Improved Agility, Flexibility and Resiliency: Use insights available from richer IT/OT data sets to more quickly adjust to production and market changes and restore production after disruptions.
- Enhanced Product Quality: Real-time quality control and process optimization, leveraging IT/OT data, minimizes defects and ensures consistent product quality, leading to increased customer satisfaction.
- Reduced Costs: Predictive maintenance minimizes repair costs and extends equipment lifespan. Optimized inventory management reduces carrying costs.
- Fostered Innovation: By providing a comprehensive view of operations and customer needs, IDM empowers manufacturers to collaborate and identify new opportunities for product development and process improvement.
By prioritizing Industrial Data Management, manufacturers can unlock the full potential of Industry 4.0 solutions, driving significant improvements in efficiency, quality, and innovation. It's not just about keeping up; it's about gaining a competitive edge in the increasingly digital world of manufacturing. And how do you know if you’re making progress with IDM – just consider whether you are making manufacturing and operations data more meaningful, useful and valuable.