A New Era of Digital Condition Based Maintenance Is Emerging in Aerospace and Defense
There is no doubt that in the past decade mega-trend technologies have altered all industries and really, life as we know it. What’s new in the technology landscape is the rapid commercial growth and use of Generative Artificial Intelligence (AI), in the form of powerful tools and services that create new data, procedures, analyses and insights and also support the broad adoption of affordable Machine Learning (ML) which is now part of the AI fabric.
So, what effect have these technological advances in AI had on a new era of Condition Based Maintenance (CBM)?
CBM+ was introduced in November 2002 by the DUSD L&MR. It was further defined in March 2004 via DoDI 4151.18 and later codified in the Condition Based Maintenance Plus DoD Guidebook, May 2008.
The industry continues to adopt this mandate and with the current advancements in CBM in August 2020 with the publication of the latest US Department of Defense instruction on, “Condition Based Maintenance Plus (CBM+) for Materiel Maintenance,” DoDI 4151.22, there is even more pressure on industries to have a level of integration that older technologies didn't even provide.
DoDI 4151.22
Issued by the Under Secretary of Defense for Acquisition and Sustainment, this instruction included an adoption mandate for all military services, agencies, research organizations and combatant commands, covering new and fielded weapon systems programs.
The key elements of this mandate include:
During the acquisition phase of new weapon systems:
CBM+ capability will be a mandatory key performance parameter and system attribute required for Acquisition Category (ACAT) I & II programs.
Specification of capabilities for:
- Condition-based and predictive maintenance models that use field operations and other data
- Optimization of material availability for timely servicing to:
- Maintain availability, operational readiness and mission capability
- Minimize unscheduled downtime and repairs
- Eliminate unnecessary maintenance actions
- Incorporate cost-effective system health management processes into operations.
For fielded weapon systems:
Improve materiel readiness and affordability for fielded systems, the Military Services and lifecycle managers will consider implementing and executing CBM+.
Comply with cybersecurity, SIM and IUID requirements in accordance with DoDI 8500.01, SIM in accordance with DoDI 4151.19, and IUID in accordance with DoDI 8320.04
CBM Maturity Roadmap
Kalypso, the digital services arm of Rockwell, has studied this DoD Instruction, and it is clear many of the global industrial markets we have been serving for decades already use our data-driven, predictive maintenance capabilities that align with the DoD’s new instruction.
Now, with Industrial Internet of Things (IIOT) platforms in routine use enabling real-time sensor/data aggregation combined with powerful AI/ML analytical processes, CBM+ functionality contemplated in the original DoD Instruction can now be significantly enhanced.
Our vision and capabilities provide the knowledge and experience to inform a complementary direction to mature CBM+ beyond the technological constraints of the original thinking and instructions to a more advanced maturity called CBM++.
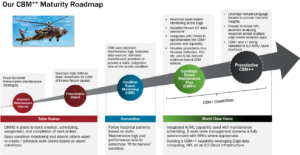
To us, the plus in a CBM+ solution today should be able to provide real-time monitoring of an asset’s health with robust application AI/ML capabilities.
Our point of view for CBM++ and its future functionalities are here today
In a CBM++ solution, we see a far broader incorporation of multi-sensor strategies into the instrumenting of fielded assets. Today’s leading Industrial Internet of Things (IIoT) strategies leverage current sensor capabilities (such as optical, non-optical spectrum and multi-modal) and the ability to stream and aggregate the raw sensor data to compute on the “Edge.” This is supported by a local, scalable and IIoT platform for analytics and visualization.
Sensor tensor fusion techniques could also be triggered through local Db clusters for AI/ML inferencing, training and tuning of predictive models with real-time results transported via MQTT/Wireless connection to a secure cloud.
This real-time solution and rich dataset can be mined for things like anomaly detection or remaining useful life predictions, all providing AI/ML-derived evidence from the CBM++ infrastructure.
The Process Steps of Prescriptive CBM++
Extending this foundational capability into what we're calling the Prescriptive CBM++ domain is all within our reach. What's even more exciting is the inclusion of a currently “dark” dataset into our CBM++ model e.g., textual data.
Imagine being able to mine the enormous reservoir of document-oriented data like maintenance logs, in-service configurations, MRO work scope, flight mission records and air worthiness alerts.
This vast unstructured data source can now be assimilated into the digital data fabric of AI/ML models not typically used in CBM analysis. The application of Natural Language Processing (NLP) techniques combined with a purpose-built AI/ML algorithms, unlocks insights previous masked from the traditional CBM processes and practices.
NLP is widely used in today’s document-intense environments like finance, law and healthcare. When correlated with other digital datasets, the results can be transformative. This is the vision we have for the new CBM (++) digital era and capability noted in our CBM Maturity roadmap graphic above.
Again, we want to acknowledge the progress that has been made today in the application of the new CBM+ mandate and offer this additional example. The Department of the Air Force Rapid Sustainment Office (RSO) in 2022 created the Predictive Analytics and Decision Assistant (PANDA) program and designated an artificial intelligence and machine learning tool for predictive maintenance. C3 ai is the software company that partnered with the AF/RSO to support an initial tool that complies with the stated requirements under the PANDA program. A point solution such as this provides a first-level capability where proof of concepts can be launched demonstrating some level of functionality which will be a good first step.
While the implementation of a point solution has merit, our view is that this approach falls far short of the needed operational viability and scaling necessary to address the critical need of asset readiness, and reliability in mission for protecting our warfighters.
In order to insert the needed CBM++ capability, either in the context of an industrial or military enterprise, one must think through the process steps required to effectively operationalize and scale the capability.
Leveraging our experience with defense clients and these technologies, we've compiled eight actions and deliverables required to standup a scalable CBM++ capability within any operation:
- Define and standardize CBM+ data models
- Create and use consistently templates that identify which equipment and adjacent system parameters are monitored for a categories of assets, and which will enable rapid scaling to additional assets or fleets
- Establish secure IT infrastructures that enable and support real-time sensor streaming and analytics
- Standup dashboards that can display real-time sensor data with key metrics and analytics defined by Command Ops
- Implement AI/ML-enabled monitoring of CBM+ data streams
- Establish a baseline of parameters for asset behavior under normal conditions
- Create a list of operationally relevant rules monitoring parameter(s) and or condition(s) that identifies a significant change indicative of a developing fault or failure
- Construct and integrate situationally aware Asset Profile and Visualization Displays
- Real-time Dashboards that display the status of all assets, including vehicle ID #, location, status and availability, etc.
- Apply advanced AI/ML capabilities to system monitoring and performance analysis
- Apply AI/ML-based detection and pattern recognition techniques for prediction of asset faults and impending failures (e.g., vibration, motor circuit analysis, oil analysis, thermography, ultrasonic and more)
- AI/ML-driven Anomaly Detection
- Develop a predictive model that provides early identification of probable faults when there is limited or no historical data
- Tune the AI/ML engine to learn patterns of potential defects and deviations from known asset baselines and absorb expert feedback in the form of labels for identified operational states
- Orchestrate Data
- Contextualize the maintenance event data, process data, and CAD assets and P&ID documents from disparate sources for full asset lifecycle analysis
- Integrate of CBM+ capabilities to Computerized Maintenance Management System (CMMS) /Enterprise Asset Management (EAM) solutions for Automated Work Order Creation
- Achieve closed loop CBM++ capability through open API integration with systems for advanced work order automation and processes from materiel management, maintenance management, etc. that together organize and execute the actions of asset sustainment
We acknowledge the progress that has been made in elevating the priority and maturity of the Conditioned-Based Maintenance Plus capability as it pertains to weapon systems for the warfighter. However, given the context of the current global threat and the fact that our weapons systems have fallen behind in readiness and capabilities, we must move faster.
This Viewpoint article is intended to move beyond point solutions and provide the blueprint for preparing to deploy and scale more advanced AL/ML-based CBM++ solutions that are here today.
What’s Next?
Part II of our Viewpoint Series addresses the need for a closed-loop operating model and framework that describes four essential process pillars to be in place to ensure that CBM++ capabilities can be connected, informed, fed with essential data provided at the right time, and utilize the digital thread from end to end. Interested in learning more about the closed-loop operating model and framework that pulls together the eight key process steps we discussed above along with the four foundational pillars we’ve identified? Stay tuned for Part II.
You can also check out Glenn’s CBM++ Fireside Chat webinar.