To solve for this complex problem, we developed a pattern recognition technique that compared new spec inputs with historical data to gauge the likelihood of an entry error.
For example, a shipping unit that is 2 feet tall is less likely to be 4 inches wide than it is to be 4 feet wide. If a user enters 4 inches, the advisor would detect that as an anomaly. By comparing the combination of the properties in a specification, the advisor is able to intelligently suggest where entries should be reviewed or modified.
A variety of techniques were used including:
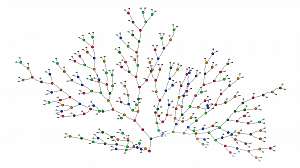
A random forest model - a collection of decision trees - was trained on historical rejections, to identify patterns in the data that point to a high likelihood of rejection.
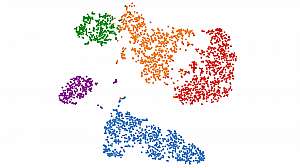
A clustering algorithm to identify anomalies present in the measured weight and dimensions of specifications. Outliers were identified based on the statistical distribution of groups of similar and comparable parts.
To maximize the utility of the AI analysis, we developed an interface that shows a heat scale to quickly communicate risk of rejection, a detailed ranking of the contributing factors to rejection and prescriptive suggestions that can be acted upon by the user.
Once the user takes action, immediate feedback is provided to signal successful risk reduction. Working through this process early in spec origination reduces the frequency of errors that result in costly downstream issues.
A quick primer on why specification management is challenging and critical to get right
- Specification management is critical to getting products from concept to store shelf. Multiple checklists or rules are used for data quality verification, which is often done manually.
- Ever-accelerating innovation timelines means people are working with increasing volumes of high complexity specifications
- When specification errors happen, for example in weight and dimension of a product, they commonly result in rework and loss of productivity. In severe cases they can trigger a cascade of financial losses through customer fines and freight costs, like overfilled or underfilled trucks, and late shipments, returns or order cancellations.
Our work created a feedback loop that builds on itself to create continuous improvement in spec quality, driving increased productivity by getting it right the first time.
Thought Leaders
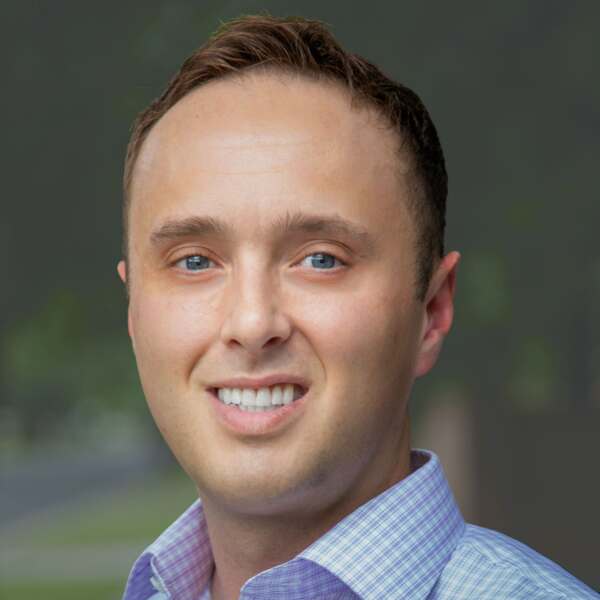
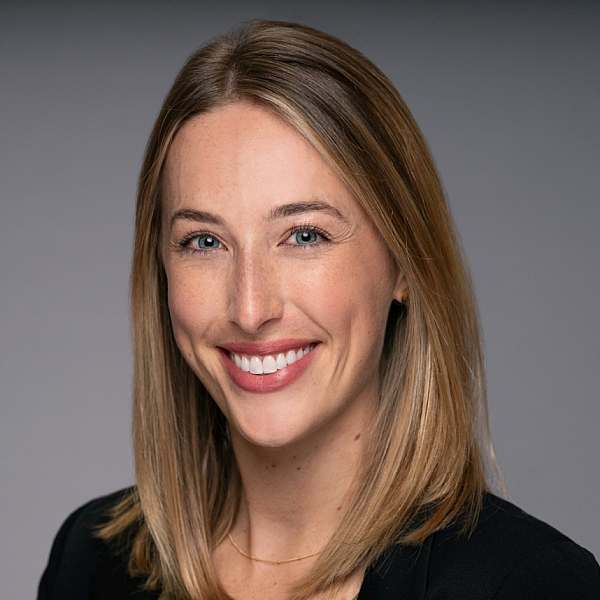