To provide a digestible and useful metric, we needed to develop a robust and quantitative comparison method and evaluate the outputs through a human lens.
We wanted a method that would allow us to navigate a vast repository of formula data and measure similarity between complex formula structures while accounting for the varying perspectives under which similarity is evaluated.
Our analysis found the best fit to be not one metric, but instead an ensemble of metrics that apply different conceptions of distance and likeness for a balanced result.
To answer for the question of context and therefore make that metric meaningful to a user, we compiled training data that was function-specific. The function-specific training data – from safety testing, regulatory, product performance, etc. – was then used to weight, or tune, the measures of distance to be specific to how that function views similarity.
In the end, rather than providing an abstract measure of distance, we provided a direct answer to the question of similarity,
"For my purpose of evaluation, this new part, x, is 95% similar to previously released part, y.
...and therefore
The safety evaluation done on part y is transferrable to part x."
With different functional contexts applied, we can answer a multitude of actionable questions:
- Is this new formula so similar to existing formulas that there is no need to repeat already conducted safety tests?
- Can regulatory clearance be expedited (because we’ve already done it with essentially the same formula)?
- Can claims statements be repurposed?
- Do prior performance tests apply? ...and so on.
The model is built to learn and fine-tune those contexts over time so the answers to those questions continue to remain relevant as business practices and policies evolve.
A quick primer on why comparing formulas is important but complicated.
In the realm of safety assessment, regulatory approvals, and product evaluations, there is a concept called "read-across", where two formulas are so alike that the testing, evaluations, and approvals done for one can be transferred to another.
In other words, several rounds of costly and time-consuming lab testing can be skipped when a nearly identical formulation has already been evaluated and approved.
The challenge is with properly identifying how "similar" those things are. Not only are formulas complex, multi-level structures, but "similar" means different things to different functions.
An example would be a cake mix. An allergist would want to know what possible allergens go in, while a baker would be interested in how those ingredients create the end product, like knowing which fats might develop an off-flavor in the cooking process.
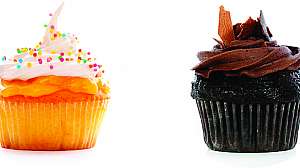
A baker would consider a chocolate cake mix to be very different from a vanilla one, while the allergist would be focused on the fact that they both contain common allergens such as dairy, wheat, eggs, and soy and to her, the two mixes may be more "similar."
Our work delivered a result that was not only analytically robust, but also user friendly – answering a highly subjective question with a simple objective measure.
We helped the client look at their data differently, and created a foundational model that is highly extensible to future opportunities related to the manipulation, analysis, and optimization of formulated products.
Thought Leaders
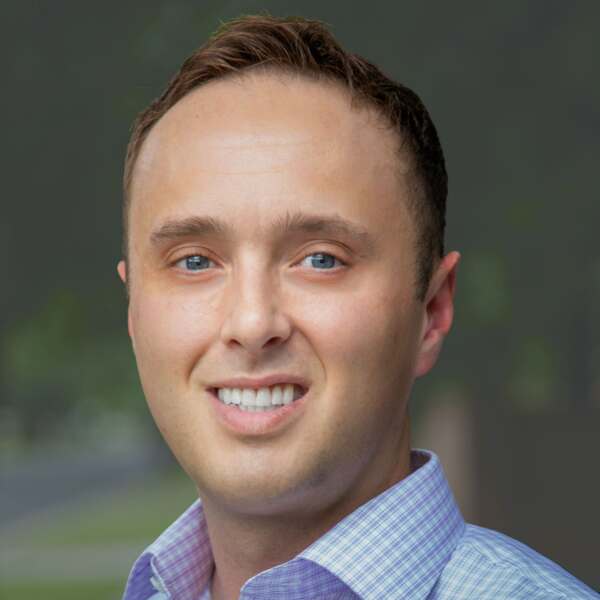
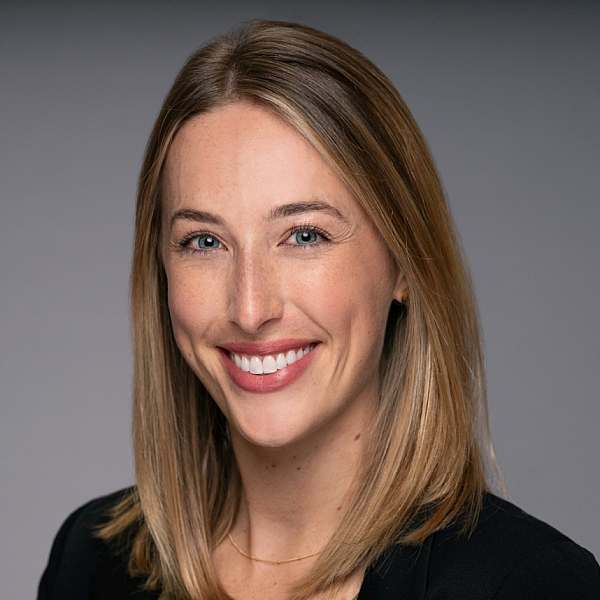